Interview with Daniel Müller-Gritschneder from TU Munich
.
The PENTA project ECOMAI started with a master thesis led by INFINEON, a world leader in semiconductor solutions. The thesis had one focus:
“How can the control of an electrical motor be significantly enhanced with an “AI” (“Artificial Intelligence”)-driven, self-learning control algorithm?”
Dr.-Ing. Daniel Müller-Gritschneder from the Technical University of Munich, Chair of Electronic Design Automation, supervised this thesis. One of his research topics focuses on TinyML, which provides methods to run Neural Network Inference on Low-power Micro-Controller Units (MCUs). As electrical motors and their control MCUs can be found in almost all applications with moving parts they are a key energy consumer. In 2011, a study has shown that electric motors consume 40% of the power consumption worldwide. Additionally, they are responsible for 20% of the worldwide CO2 emission. Following up on the initial results from the master thesis, the question arose whether tinyML can provide an AI-enhanced motor control solution, which can be translated into a gamechanger from an economic and ecological perspective in terms of energy consumption of electrical motor drives. With that the idea of the European PENTA project ECOMAI was born.
From the Master Thesis to the Vertical Project ECOMAI
The development of novel motor control MCUs up to electrical motor drive systems is managed by different kind of experts, in scientific organizations as well as in research departments in the tech industry. Yet due to the convincing initial results, it was quite easy to gather the different parties needed together fort he project proposal. “After we came to the point to gather a significant consortium, we were in the position to clearly state the project scope: Applying Tiny ML for AI-enhanced Motor Control and conditional monitoring is not only the goal of our industry partners, which come from different industries, but also from our scientific partners. We also have technology partners on board, who can deliver technical tools and deliver a development framework to build the future solution upon”, says Daniel, the project technical lead from TU Munich.
ECOMAI with its vertical project structure includes 11 consortium members from Germany, Austria and Turkey. They are bringing together profound expertise in hardware and MCU design, tinyML, electrical motor drive system design and predictive maintenance.
Translating research into a meaningful business opportunity
With partners ranging from research institutions to suppliers of end products, the project’s innovations will have the potential to move quickly into commercial applications. Daniel explains the strength of the consortium. “We defined several use cases for ECOMAI in order to reach our milestone 1. We shared our knowledge and expertise between the different technical field experts to define the use cases as meaningful and result-oriented as possible. This is a critical phase in this kind of project and it always takes some time. But it is the key to developing application-oriented outcomes, which will work in an industrial setting. Having members on board covering the entire development cycle aids in our efforts and shows the strong interest in the project result, ”Daniel continues. With ECOMAI, Daniel and the research organizations involved demonstrate, that scientific research delivers more than basic answers but delivers a valuable contribution to both sides: the economic as well as the ecological questions of our demanding time.
The Vision of lowering energy consumption of electrcial drive systems by delivering an AI solution
The use cases of ECOMAI are very practical-oriented and are the result of a vision process which always had the end-use in mind. They include electrical motors in automotive compressor system, in the screen doors found in trains stations as well as in an rehabilitation robot. A better customer experience by increasing the uptime and lifetime of these systems by predictive maintenance, cost-efficiently implemented by TinyML near the motor, will improve the daily life from all of us. At the same time, TinyML will be used to save energy from one of the most energy consumers, electrical motors, by providing AI-enhanced motor control algorithms:. Daniel explains: “For me, the motivation behind ECOMAI is also, to actively shape the technological change. Energy efficiency has a significant impact on our life. Together with our expertise and know-how of the consortium and thanks to the support of our multinational funding partners we are in the position to strive for the vision of ECOMAI.”
About Dr.-Ing. Daniel Müller-Gritschneder
Technical University of Munich, Chair of Electronic Design Automation (Prof. Schlichtmann)
Daniel Mueller-Gritschneder is currently holding the position of Adjunct Teaching Professor (Privatdozent) for Electronic System Level Design Methods at the Institute for Electronic Design Automation (Prof. Schlichtmann). He was the temporary acting head of the Chair of Real Time Systems (www.ei.tum.de/rcs) from Nov. 2019 until March 202. He received his Diploma and doctoral degree in electrical engineering and information technology from TUM in 2003 and 2009 respectively. In 2019 he completed his habilitation at TUM. He is senior member of IEEE. He received the best paper award at SiPS 2019, the Habilitation Award of the Bund der Freunde der technischen Universität Muenchen in 2019, the 2nd best paper award at SMACD’15, and his papers were nominated for best paper at DAC’07, DATE’10, Analog’10 and NOCS’13.
* Data Source: D. W. E. Dr. Patrick Plötz, „Zukunftsmarkt Effiziente Elektromotoren (German), Fraunhofer Institut für System- und Innovationsforschung (ISI),“ 10 2011.
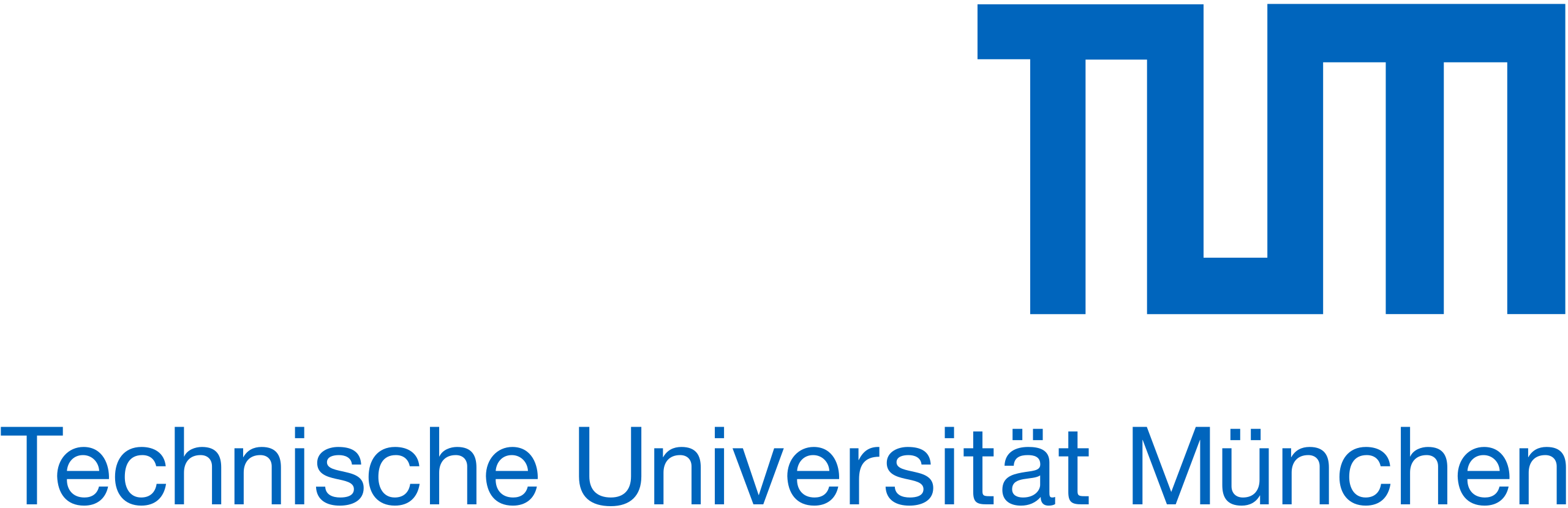
“The future of AI is tiny. We are very curious to research new ideas in the ECOMAI project how tinyML can be used to reduce the energy of electrical motors with our partners. This can have big impact on sustainability in the future as electrical motors are used everywhere.”